Leveraging Machine Learning Development Services for Predictive Maintenance in Manufacturing
Explore how machine learning development services revolutionize predictive maintenance in manufacturing. Enhance equipment reliability, reduce downtime, and optimize operations with advanced AI-driven insights and proactive maintenance strategies.
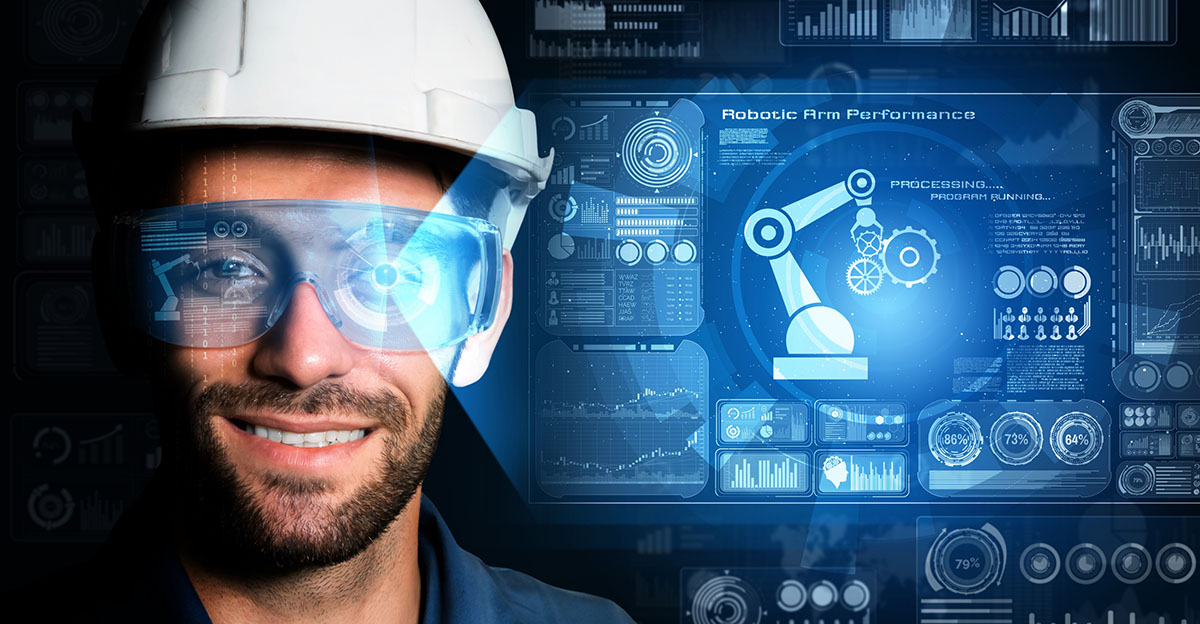
In the fast-paced world of manufacturing, operational efficiency and cost-effective maintenance are essential to maintaining competitiveness. Predictive maintenance powered by machine learning (ML) is revolutionizing how manufacturers approach equipment maintenance, offering significant improvements in efficiency and reducing the risk of unexpected downtime. In this blog, we'll explore how machine learning development services are transforming the manufacturing industry, particularly in the area of predictive maintenance.
What is Predictive Maintenance?
Predictive maintenance refers to the use of technology to predict when equipment will fail so that maintenance can be performed just before failure occurs. This method contrasts with traditional maintenance strategies, like reactive or preventive maintenance, where equipment is either fixed after it fails or maintained at scheduled intervals regardless of its condition. Machine learning for manufacturing takes this a step further, leveraging data to forecast equipment malfunctions based on real-time usage patterns.
The Role of Machine Learning in Predictive Maintenance
1. Data-Driven Insights
One of the key factors driving the adoption of predictive maintenance is the ability to collect and analyze vast amounts of data. Machine learning development services play a pivotal role by applying algorithms to this data to detect patterns and anomalies in equipment performance. For example, temperature readings, vibration levels, and other sensor data are continuously monitored, and machine learning models process this information to predict when a machine is likely to fail.
2. Anomaly Detection for Early Warning
A significant benefit of machine learning in predictive maintenance is its ability to detect anomalies in equipment behavior that might signal an impending issue. AI-driven equipment monitoring systems can identify small deviations from normal operations, allowing maintenance teams to take preventive measures before the problem worsens. For example, a slight increase in motor temperature could be an indicator of an impending failure, and predictive maintenance tools can trigger alerts for timely intervention.
3. Automation of Maintenance Tasks
AI-powered systems can automate diagnostics and even schedule maintenance, which significantly reduces human error and intervention time. Machine learning models, when integrated with smart factories, automatically analyze equipment health and prioritize maintenance activities based on urgency. This automation can also support mobile app development company efforts by allowing technicians to receive real-time updates and alerts via mobile applications, improving on-site response times.
How Machine Learning Benefits Manufacturers
1. Increased Uptime
Machine learning enables manufacturers to address equipment problems before they result in unplanned downtime. This proactive approach ensures that manufacturing lines are operational for longer periods and that production targets are met consistently. Manufacturers that implement predictive maintenance strategies often experience a significant reduction in downtime.
2. Cost Reduction
The implementation of predictive maintenance can lower operational costs by reducing unplanned repairs and unnecessary maintenance. Rather than spending resources on scheduled maintenance that isn’t needed, companies can focus on maintaining equipment only when it's necessary. This strategy can lead to lower repair costs and extend the lifespan of expensive machinery.
3. Better Decision Making
With the ability to analyze vast amounts of data from connected devices, manufacturers can gain deeper insights into their operations. These insights enable more informed decision-making regarding asset management, repair schedules, and resource allocation. As machine learning for manufacturing continues to evolve, the use of real-time data will become an integral part of every factory’s decision-making process.
4. Enhanced Operational Efficiency
By integrating predictive maintenance solutions into daily operations, manufacturers can streamline their workflows. Predictive analytics helps in avoiding the downtime caused by machine failures, ensuring smooth and continuous production. Automated insights from machine learning systems also support real-time operational adjustments, boosting efficiency on the factory floor.
Practical Applications of Machine Learning in Predictive Maintenance
1. Smart Inventory Management
Machine learning also plays a significant role in managing spare parts and inventory. By analyzing past maintenance history and equipment usage, machine learning algorithms can predict when spare parts will be needed and optimize inventory management. This minimizes inventory costs and ensures that the right parts are available when necessary.
2. Automated Pricing Strategies
AI-driven solutions are not limited to maintenance; they can also assist in dynamic pricing strategies. Machine learning can analyze market conditions, inventory levels, and demand forecasts to adjust pricing in real time. Predictive maintenance data can also inform pricing decisions, as the anticipated longevity of equipment can influence the cost-effectiveness of certain machines or services.
3. AI-Powered Customer Service
Manufacturers often face customer inquiries related to equipment issues or product delays. AI-powered chatbots can be integrated into predictive maintenance systems to provide real-time updates to customers. AI Chatbot Development Services allow manufacturers to deliver instant responses to customers regarding the status of equipment or orders, improving customer satisfaction and engagement.
How to Get Started with Machine Learning for Predictive Maintenance
1. Partner with a Trusted AI Development Company
To successfully implement machine learning-based predictive maintenance, manufacturers should work with an experienced Generative AI development services provider. A reliable development partner will assist with data integration, machine learning model development, and the deployment of AI-powered monitoring tools. These experts ensure that your predictive maintenance system is tailored to your specific needs and challenges.
2. Invest in IoT Infrastructure
The backbone of predictive maintenance systems is the data generated by sensors and Internet of Things (IoT) devices. Smart factories require a network of connected devices to monitor equipment performance in real time. Manufacturers must invest in IoT infrastructure, including sensors, communication protocols, and cloud computing, to collect and analyze data efficiently.
3. Utilize Mobile Applications for Maintenance Teams
As part of the digital transformation, mobile app development companies can help develop mobile solutions that enable maintenance teams to access equipment data on the go. These applications can provide real-time alerts, diagnostics, and maintenance schedules, ensuring that teams can respond quickly to emerging issues.
4. Start with Pilot Projects
Before implementing predictive maintenance across the entire organization, it is recommended to start with a small-scale pilot project. By focusing on a single machine or a specific production line, manufacturers can evaluate the effectiveness of machine learning algorithms in real-world scenarios and fine-tune the models before scaling up to other operations.
Real-World Impact of Predictive Maintenance
Companies that have implemented machine learning for manufacturing are already seeing significant results. A major industrial manufacturer that adopted predictive maintenance tools powered by AI and IoT sensors reported a 40% reduction in unexpected breakdowns and a 25% reduction in maintenance costs within the first year. Moreover, technicians were able to focus on high-priority issues, thanks to automated diagnostics and alerts.
One mobile app development company partnered with a manufacturer to create a mobile application that integrated with predictive maintenance systems. This app provided real-time alerts and maintenance schedules to technicians, improving response times and enhancing overall plant efficiency.
Conclusion
Machine learning development services are reshaping the way manufacturers approach equipment maintenance. By leveraging AI-driven tools for predictive maintenance, companies can minimize downtime, extend the lifespan of their equipment, and optimize operational efficiency. As the manufacturing industry continues to embrace digital transformation, the implementation of machine learning for predictive maintenance will become a key factor in maintaining competitiveness.
To harness the full potential of these technologies, manufacturers should partner with reliable Generative AI development services providers, invest in smart infrastructure, and start small with pilot projects. With the right tools and expertise, predictive maintenance powered by machine learning can lead to significant cost savings and operational improvements.
FAQs About Machine Learning for Predictive Maintenance
How Does Machine Learning Enhance Predictive Maintenance in Manufacturing?
Machine learning models can analyze historical and real-time sensor data to detect patterns and predict when a machine might fail. This proactive approach allows manufacturers to perform maintenance only when necessary, avoiding costly downtime and repairs.
What Are the Benefits of AI-Powered Equipment Monitoring?
AI-driven equipment monitoring provides real-time insights into machine health and alerts maintenance teams to potential issues before they result in failures. This leads to more efficient use of resources, increased uptime, and reduced costs.
Can Machine Learning Help Reduce Downtime in Manufacturing?
Yes. By predicting when machines will fail, machine learning systems allow manufacturers to perform maintenance in advance, preventing unplanned downtime and minimizing disruptions to production.
What's Your Reaction?
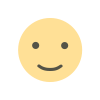
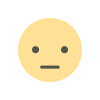


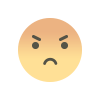
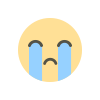
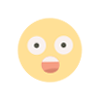